Fundamentals of Statistics
Completion requirements
View
3. Type I and Type II Errors
An experiment testing a hypothesis has two possible outcomes: either H0 is rejected or it is not. Unfortunately, as this is based on a sample and not the entire population, we could be wrong about the true treatment effect. Just by chance, it is possible
that this sample reflects a relationship which is not present in the population – this is when type I and type II errors can happen.
All statistical hypothesis tests have a probability of making type I and type II errors. For example, blood tests for a disease will erroneously detect the disease in some proportion of people who don't have it (false positive), and will fail to detect
the disease in some proportion of people who do have it (false negative).
Type I - when you falsely assume that you can reject the null hypothesis and that the alternative hypothesis is true. This is also called a false positive result. A type I error (or error of the first kind) is the incorrect rejection
of a true null hypothesis. Usually a type I error leads one to conclude that a supposed effect or relationship exists when in fact it doesn't. Examples of type I errors include a test that shows a patient to have a disease when in fact the patient
does not have the disease, or an experiment indicating that a medical treatment should cure a disease when in fact it does not. Type I errors cannot be completely avoided, but investigators should decide on an acceptable level of risk of making type
I errors when designing clinical trials. A number of statistical methods can be used to control the type I error rate.
Type II error - when you falsely assume you can reject the alternative hypothesis and that the null hypothesis is true. This is also called a false negative result. A type II error (or error of the second kind) is the failure to reject a false
null hypothesis. This leads to the conclusion that an effect or relationship doesn't exist when it really does. Examples of type II errors would be a blood test failing to detect the disease it was designed to detect, in a patient who really has the
disease; or a clinical trial of a medical treatment concluding that the treatment does not work when in fact it does.
|
Null hypothesis is true |
Null hypothesis is false |
Reject the null hypothesis |
Type I error |
Correct outcome |
Fail to reject the null hypothesis |
Correct outcome |
Type II error |
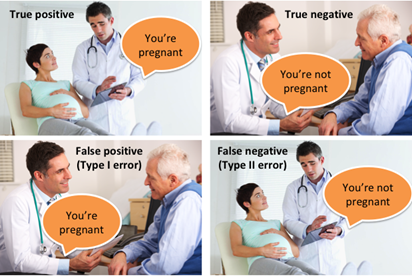