Fundamentals of Statistics
Site: | EUPATI Open Classroom |
Course: | Statistics |
Book: | Fundamentals of Statistics |
Printed by: | Guest user |
Date: | Thursday, 3 April 2025, 11:46 AM |
1. Purpose and Fundamentals of Statistics
(This section is organised in the form of a book, please follow the blue arrows to navigate through the book or by following the navigation panel on the right side of the page.)
Data alone is not interesting. It is the
interpretation of the data that we are really interested in…
Jason Brownlee – 2018
Statistical methods provide formal accounting for sources of variability in patients’ responses to treatment. The use of statistics allows the clinical researcher to form reasonable and accurate inferences from collected information, and sound decisions in the presence of uncertainty. Statistics are key to preventing errors and bias in medical research.
Hypothesis
testing is one of the most important concepts in statistics because it is how
you decide if something really happened, or if certain treatments have positive
effects or are not different from another treatment. Hypothesis testing is
common in statistics as a method of making decisions using data. In other
words, testing a hypothesis is trying to determine if, based on statistics, your
observations are likely to have really occurred. In short, you want to proof if
your data is statistically significant and unlikely to have occurred by chance
alone. In essence then, a hypothesis test is a test of significance.
- Null and alternative hypothesis
- Sample size
- Bias
- Type I error
- Type II error
- Significance
- Power
- Confidence intervals
2. What Is Hypothesis?
2.1. Null and Alternative Hypothesis
- The null hypothesis (H0): there is no difference between the existing standard of care treatment ‘A’ and the new treatment ‘B’ and any observed differences in outcome measures are due to chance.
- The alternative hypothesis (H1): the new treatment ‘B' is better than the existing standard of care treatment ‘A’.
2.2. What Is Hypothesis Testing?
3. Type I and Type II Errors
|
Null hypothesis is true |
Null hypothesis is false |
Reject the null hypothesis |
Type I error |
Correct outcome |
Fail to reject the null hypothesis |
Correct outcome |
Type II error |
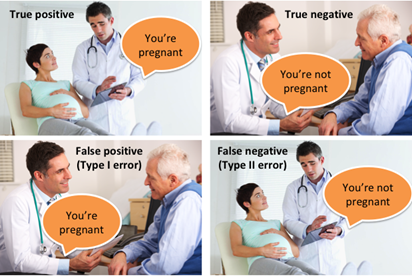
4. Power of a Statistical Test
5. Sample Size
- Magnitude of the expected effect.
- Variability in the variables being analysed.
- Desired probability that the null hypothesis can correctly be rejected when it is false.
5.1. Sampling Error
6. Significance Level
- a large p-value suggests that the observed effect is very likely if the null hypothesis is true.
- a small p-value (equal to or less than the significance level) suggests that the observed evidence is not very likely if the null hypothesis is true – i.e. either a very unusual event has happened or the null hypothesis is incorrect.
7. Confidence Interval
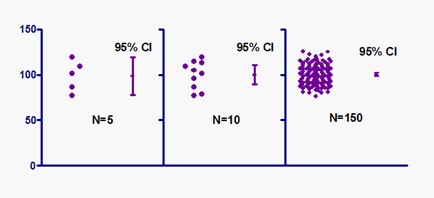
Figure 1: Effect of increased sample size on precision of confidence interval (source https://www.simplypsychology.org/confidence-interval.html)
Confidence intervals (CI) provide different information from what arises from hypothesis tests. Hypothesis testing produces a decision about any observed difference: either that it is ‘statistically significant’ or that it is ‘statistically nonsignificant’. In contrast, confidence intervals provide a range about the observed effect size. This range is constructed in such a way as to determine how likely it is to capture the true – but unknown – effect size.
The following graphs exemplify how the confidence interval can give information on whether or not statistical significance for an observation has been reached, similar to a hypothesis test:
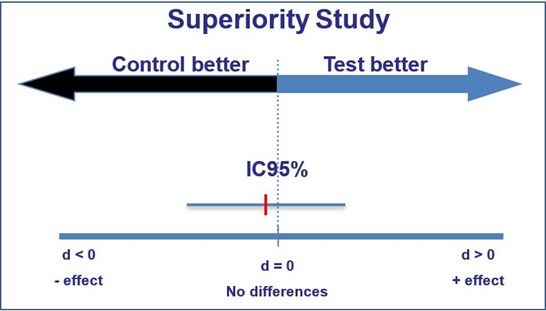
If the confidence interval captures the value reflecting ‘no effect’, this represents a difference that is statistically non- significant (for a 95% confidence interval, this is non-significance at the 5% level).
If the confidence interval does not enclose the value reflecting ‘no effect’, this represents a difference that is statistically significant (again, for a 95% confidence interval, this is significance at the 5% level).
In addition to providing an indication of ’statistical significance’, confidence intervals show the largest and smallest effects that are likely, given the observed data, and thus provide additional useful information.