1. Data sources for pharmacoepidemiological research
1.4. Patient-Generated Health Data (PGHD)
Patient-Generated Health Data (PGHD) [1]
"The amount of health information generated digitally across socio-cultural domains is unprecedented." The Committee on Data for Healthy Societies [2]
With the rapid rise of digital health, encompassing electronic healthcare data sources, such as electronic health records (EHR) and administrative/claims databases and further advanced by the development of innovative, often mobile, digital health technologies, the landscape of health care and related research has changed considerably.
Without going into further detail, the following graph gives an overview of the basic components of digital health in the healthcare ecosystem.
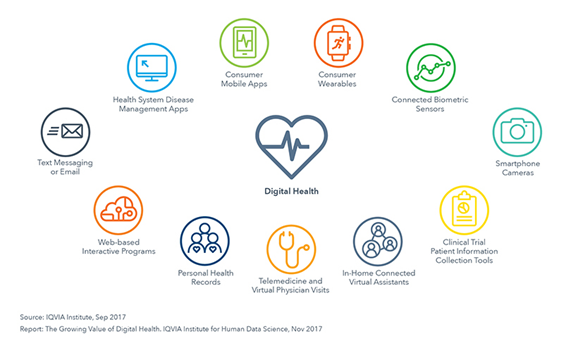
Figure 1: Non-comprehensive overview of digital health components.
This is accompanied by an emerging emphasis on patient centricity highlighting the growing importance of Patient (or Citizen/Person) Generated Health Data (PGHD) as a new data source which can be positioned on the intersection between the digital revolution and the patient-centred care movement. PGHD has made it possible for patients to capture, use, and share their health data in day-to-day settings and in real time with clinicians and researchers.
In the past, researchers often were limited to the data collected at the study site at regular intervals or from logs of data captured by patients and brought to the study site. With PGHD, new types of data are available in increased volume and frequency directly from patients using digital devices, both passively [4] and actively [5]. For example, health devices, such as connected glucose sensors, record data at short intervals or continuously, and they can transmit data directly and electronically to patients themselves and to clinicians and researchers.
Figure 2 highlights some of the sources and tools that facilitate collection of PGHD. There may be overlap between tools, such as when smartphones are used to complete online surveys, or their built- in sensors facilitate passively collected data.
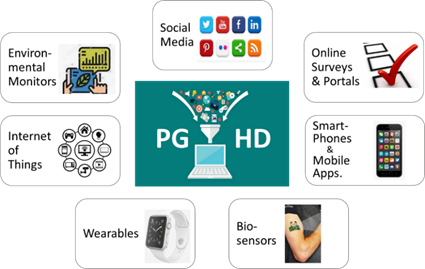
Figure 2: sources and tools for collecting PGHD (after Bourke et al.,2020)
At the population level, researchers can analyse large volumes of PGHD using artificial intelligence (AI) and sophisticated analytical tools, can interpret free-text (applying NLP), unstructured PGHD and link them with other health-related data. Through this process, researchers can identify and confirm association between a medicine and exposure, side effects and adverse events and predict and track the spread of infectious diseases or predict outbreaks (e.g., influenza) earlier and with greater accuracy than with traditional methods. The use of PGHD technologies to capture and transmit data electronically helps to simplify the research workflow: manual data entry can be replaced by electronic data transmission into a research Findings database, data checking for completeness and preparation for analysis can likewise be automated and the potential for human error during data entry can be minimised.
Specifically, for pharmacoepidemiology PGHD has the potential to address some of the existing challenges such as misclassification, representativeness, and missing information. It helps to gain person-centric insight not readily available in routine healthcare datasets and collect more and different variables, and with greater frequency/continuity than may be possible in clinical/research settings. In pharmacoepidemiology and epidemiology research studies, PGHD can be viewed as complementary, rather than a replacement of other data sources.
The following lists a number of possible advantages and challenges (not comprehensive) when applying PGHD data in healthcare and pharmacoepidemiologic research.
Advantages of PGHD
- empower the patient to track, change and improve health by enabling better self-management
- support healthcare professionals monitor and assist their patients, allowing real-time adjustments to treatment in response to patients' symptoms and physiology
- improve relationships and communication between patients and healthcare teams and support shared decision-making
- augment patient-driven quality of care assessment
- provide additional information to inform pharmacoepidemiologic research on:
o adverse events, especially non-serious events, their severity or their impact on patients' lives
o previously unassessed factors including patient-reported outcomes such as functionality, quality of life, pain or depression scales, as well as more accurate and precise information on timing and severity
o additional covariates (potential confounders and effect modifiers) which may not be present in EHRs, such as weight, smoking, alcohol consumption, as well as physical activity, sleep, mobility, location, diet and biochemistry (e.g., home-based blood glucose measurements).
Challenges of PGHD
- Imbalanced penetration among the entire population
- underdeveloped strategies for long term patient engagement
- data validity – e.g., devices to objectively measure medicines use
- data generalisability – especially relevant when using social media data
- concerns that receiving PGHD adds to clinical workloads and disrupts workflows contrary to expectations
- paucity of validated instruments, e.g., to measure patient reported outcomes.
- device standardisation and consistent accuracy
- high volume data is challenging to analyse because of its format or high frequency of measurement (e.g., continuous recording of heart rates or blood pressures).
- technical and privacy concerns, cybersecurity - can make linking disparate data sources problematic
- legal aspects such as informed consent and data security, (electronic-consent (e-consent))
- possible third-party involvement for encryption, pseudonymizing and anonymization for security of sensitive data.
- selection bias is possible if patient characteristics that underlie the ability to create PGHD (e.g., ownership of certain digital devices) are associated with both exposure and outcome of interest
Examples:
- built- in sensors in digital devices facilitate passively collected data, e.g., accelerometry to estimate hand tremors in people with Parkinson's Disease
- Smartphones: collection of unique types of PGHD, such as speech recordings in assessment of Parkinson's Disease severity
- Smartphones: frequency of social communication to monitor episodes of depression
- Digital Health Applications (DiGAs)[6], authorised by regulatory authorities are on the rise. A DiGA can not only include software but also devices, sensors (and other hardware such as wearables). A few examples from the DiGA directory of the German Federal Institute of Drugs and Medical Devices (BfArM)[7], the first regulatory authority to approve DiGAs, are given in the table below together with their indication (status 04/2021):
Name |
|
deprexis |
depressive episodes |
elevida |
Multiple sclerosis |
Kalmeda |
Tinnitus |
Rehappy |
Transitory cerebral ischemia |
somnio |
Insomnia |
zanadio |
Obesity |
[1] The following section on PGHD is mainly based on published information from the white paper of the ONC and the paper by Bourke et al.: Alison Bourke, William G Dixon, Andrew Roddam, Kueiyu Joshua Lin, Gillian C Hall, Jeffrey R Curtis, Sabine N van der Veer, Montse Soriano-Gabarró, Juliane K Mills, Jacqueline M Major, Thomas Verstraeten, Matthew J Francis, Dorothee B Bartels, Incorporating patient generated health data into pharmacoepidemiologic research Pharmacoepidemiol Drug Saf (Pharmacoepidemiology and drug safety) [2020, 29(12):1540-1549]
[4] Passive data collection: people making no or little additional effort, such as wearing/ carrying a device, or by re-using person-generated data previously recorded for non-health purposes (Examples: data from sensors in wearable and mobile devices (e.g., accelerometers, gyroscopes, GPS) or from social media (i.e., person-generated data that was initially recorded for non-health purposes providing information on exposure, confounders or outcomes).
[6] According to the German Regulatory authority (BfArM) a DiGA is a CE-marked medical device that has the following properties:
- Medical device of the risk class I or IIa (according to the Medical Device Regulation (MDR) or the transitional Medical Device Directive (MDD)). Information as to "when is an App a medical device?"; can be found here .
- The main function of the DiGA is based on digital technologies.
- The medical purpose is mainly achieved by way of its digital function.
- The DiGA supports the recognition, monitoring, treatment or alleviation of diseases or the recognition, treatment, alleviation or compensation of injuries or disabilities.
- The DiGA is used by the patient alone or by patient and healthcare provider together.
These requirements are defined in Section 33a of the German Social Code Book.
[7] DiGA directory of the German Federal Institute of Drugs and Medical Devices (BfArM): BfArM - Digital Health Applications (DiGA)