8. Data sources for pharmacoepidemiological research
Site: | EUPATI Open Classroom |
Course: | Epidemiology and Pharmacoepidemiology |
Book: | 8. Data sources for pharmacoepidemiological research |
Printed by: | Guest user |
Date: | Friday, 4 July 2025, 5:55 AM |
1. Data sources for pharmacoepidemiological research
(This section is organised in the form of a book, please follow the blue arrows to navigate through the book or by following the navigation panel on the right side of the page.)
Sources of pharmacoepidemiological data
There are two main approaches for data collection: collection of data specifically for a particular study or purpose (‘primary data collection’) or use of data already collected for another purpose, not aimed at research (‘secondary use of data’). Secondary data usually were once primary data but become secondary when used for a purpose different from the original one.
Primary data collection involves obtaining data de novo (i.e., new data), for example applying different study designs such as a prospective cohort study, surveys or randomised controlled trials. For some conditions, case-control surveillance networks have been developed and used for selected studies and for signal generation or clarification. Primary data in general offer increased control over the type and amount of information that is available as compared to secondary data. One of the limitations of primary data is the expenditure, labour and time involved in investigations with a progressive increase in sample size [1]
Secondary use of data has become the common approach in pharmacoepidemiology due to the increasing availability of electronic health records (EHR), administrative databases and other already existing data sources (see Secondary data) and due to its increased efficiency and lower cost. In addition, networking between centres active in pharmacoepidemiology and pharmacovigilance is rapidly changing (increasing) in Europe, both in terms of networks of data as well as of researchers who can contribute to a particular study with a specific data source (see Health Database networks).
In the following, brief descriptions of selected data sources in pharmacoepidemiology according to their classification are given.
[1] Harpe, S.E., Yang, Y., West-Strum, D., 2011. Using secondary data in pharmacoepidemiology. In: Understanding Pharmacoepidemiology. McGraw Hill Professional, USA.
1.1. Primary data
1. Surveys
A survey is the collection of data on knowledge, attitudes, behaviour, practices, opinions, beliefs or feelings of selected groups of individuals, by asking them in person, on paper, by phone or online. They generally have a cross-sectional design, but repeated measures over time may apply for trends assessment. If, for example, the information about specific medication-taking behaviour is required, the participant in a study that uses primary data collection can be asked. This information would most likely not be obtained through secondary data from prescription records provided by a pharmacy or routine data collections.
Survey limitations may be low response rates, allowing for possible bias. The response rate should therefore be reported in a standardised way in surveys to allow comparisons. The increasing use of online surveys requires that survey methods are adapted but should not sacrifice representativeness by accessing only populations which visit these websites (avoid bias). Similarly, the increasing use of health care professional and patient panels needs to guard against bias introduced by accessing only self-selected participants in these panels.
Example: The information from the Nord-Trøndelag Health Study from Norway as one of the largest studies ever performed is especially suitable for epidemiologic studies on blood pressure, diabetes, quality of life, tuberculosis, and other lung diseases; along with data on cardiovascular diseases, lifestyle, disablement, work, and the working environment. https://www.ntnu.edu/hunt
Randomised clinical trials follow an
experimental design that involves primary data collection. An essential
guideline on clinical trials is the European Medicines Agency (EMA) Guideline for good clinical practice E6(R2), which specifies obligations for the conduct of clinical trials to
ensure that the data generated in the trial are valid. From a legal
perspective, the Volume 10 of the Rules Governing Medicinal Products in the
European Union contains all guidance
and legislation relevant for conduct of clinical trials. (see also Randomised controlled trials (RCT) ).
A number of documents are under revision. The way clinical trials are
conducted in the European Union (EU) will undergo a major change when the Clinical Trial Regulation (Regulation (EU) No 536/2014) will fully come into effect and will
replace the existing Directive 2001/20/EC. Envisaged 2021/2022.
Internationally, a few countries support the use of national health data for research purposes, which can provide extensive information like the prevalence of smoking and other health- related risk factors, obesity among other health ailments, access to health care, etc [1].
Examples: The National Minimum Data Set (NMDS) in New Zealand is a national collection of public and private hospital discharge information, including coded clinical information, for inpatients and day patients.
The National Cohort in Germany is a government-coordinated study collecting interview and self-completion questionnaires, several medical examinations and the collection of biological material (blood, urine, saliva, nasal swabs and stool from 100.000 women and 100.000 men, including a follow up assessment of all participants after 4 years. This will provide also molecular data on a broad scale (www.nako.de).
[1] Cox, S., 2016.
Annual Update of Key Results 2015/16: New Zealand Health Survey. Ministry of
Health, Wellington
and
Gliklich, R.E., Dreyer, N.A., Leavy, M.B., 2014. Registries for Evaluating Patient Outcomes: A User’s Guide [Online]. USA. Available from: https://www.ncbi.nlm.nih.gov/books/
1.2. Registries
The term registry is defined both as recording or registering and as the record or entry itself. In epidemiology it designates the data concerning all cases of a particular disease or similar health relevant condition in a defined population so that the cases can be related to a population base. Features of a well-designed registry focus on system specifications in order to ensure continuous, efficient and collaborative data collection, safe data hosting and availability of retrievable, interoperable and re-usable data.
Registries can be used as a source of participants for studies based on either primary data collection (where the items of interest for the study are collected directly from the patients, caregivers, healthcare professionals) or secondary use of data already collected (e.g., analogous to the use of electronic healthcare records). For this purpose, registry data can be complemented with additional information on outcomes, lifestyle data, immunisation or mortality information obtained from linkage to existing databases such as national disease registries, prescription databases or mortality records. A registry-based study often uses a patient registry (see below) for patient recruitment and data collection.
Registries are further useful for:
- improvement of patient management and record-keeping
- evaluation and planning of healthcare services
- insights into risk factors for adverse outcomes
- documentation of the effectiveness of therapies in real-world settings
- provision of insight into the nature of diseases and benefit of treatments in subgroups of patients
- and provision of comparative benchmark reports to a variety of stakeholders.
When utilizing existing registries in pharmacoepidemiology research, it is important to observe the following factors which affect the value and validity of registries and databases: (1) the comprehensiveness of registration of all individuals under study and the registered data, (2) the size of the data source, (3) the registration period in order to relate the exposure and effect to possible induction and latent periods, (4) data availability, accessibility, and expenses, (5) data format, and (6) the possibilities of linkage with other data sources. The validity may also vary between different settings, and validation studies are therefore essential when using registries as a data source.
Sørensen, H.T., Johnsen, S.P., Nørgård, B., 2009. Methodological issues in using prescription and other databases in pharmacoepidemiology. Norsk Epidemiol. 11 https://www.researchgate.net/publication/41758414_Methodological_issues_in_using_prescription_and_other_databases_in_pharmacoepidemiology
Types of registries
Different types of registries exist, some are listed below:
Clinical registries gather a defined minimum dataset from individuals undergoing a particular procedure, diagnosed with a disease or utilizing any healthcare resource, and capture data systematically from existing administrative databases, medical records or directly from clinical staff using data collection forms (Hoque et al., 2016).
Patient registries are often integrated into routine clinical practice with standardised, systematic and sometimes automated data capture in electronic health records, using observational methods for the recording of data on individual patients identified by a characteristic or an event, for example the diagnosis of a disease (disease registry), the occurrence of a condition (e.g., pregnancy registry), or a molecular or a genomic feature. They can provide a real-world view of clinical practice, medication safety, patient outcomes, and comparative effectiveness. They can be viewed as a collection of patient records on a particular subject.
Disease registries, as a special form of patient registry, contain data of patients who have or have had a disease or condition of interest. A disease registry is a tool used for tracking clinical care and outcomes of a defined patient population. Disease registries can also provide flexibility for collecting and reporting data from multiple data sources. Data collection can be facilitated either by merging with another data source such as administrative data, or by survey or interview methods.
Clinical quality registries (CQRs), initially coming from Sweden, they are described as clinical registries designed to improve the quality and safety of care. CQRs are defined as “An automated and structured collection of personal data initiated with the purpose to systematically and continuously develop and safeguard quality of care.”
Population registries, e.g., those in European Nordic countries, provide a comprehensive repository of data for a high proportion or all of the population allowing linkage between government-administered patient registries that may include hospital encounters, diagnoses and procedures, such as the Norwegian Patient Registry, the Danish National Patient Registry or the Swedish National Patient Register. They may however lack information on lifestyle factors, patient-related outcomes and laboratory data.
Special populations Registries Special populations can be identified based on specific characteristics, such as age (e.g., paediatric or elderly), pregnancy status, renal or hepatic function, race, or genetic differences. Some registries are focused on these particular populations; examples are:
- birth registries
- pregnancy registries (the Systematic overview of data sources for Drug Safety in pregnancy research for an inventory of pregnancy exposure registries)
- rare diseases registries (the European Platform on Rare Diseases Registration (EU RD Platform) for information on registries for rare diseases)
- the European network of registries for the epidemiologic surveillance of congenital anomalies (EUROCAT)
- paediatric registry (Pharmachild captures children with juvenile idiopathic arthritis undergoing treatment with methotrexate or biologic agents)
- Other registries that focus on special populations can be found in the ENCePP (European Network of Centres in Pharmacoepidemiology and Pharmacovigilance) Inventory of data sources.
1.3. Secondary data
Secondary data refers to data already collected for another purpose, such as for a previous research (e.g., clinical trial), or to facilitate a process (e.g., hospital discharge records) and are often captured in an automated fashion, e.g., electronically. Although data from RCTs are not frequently used in pharmacoepidemiology, they can be used for secondary analyses. Over the last decades key data resources, expertise and methodology have been developed that allow use of such data for pharmacoepidemiology and pharmacovigilance (for more information see the Homepage | HMA-EMA Catalogues of real-world data sources and studies). These can be further linked to prospectively collected medical and non-medical data.
The key limitation of secondary data is that the data are not collected for answering the primary research question; thus, there may be specific validity issues that must be considered. They include: completeness of data capture, bias in the assessment of exposure, outcome and covariates, variability between data sources and the impact of changes over time in data, access methodology and the healthcare system.
Administrative data, claims data, data in electronic medical records and other data from digital health sources are predominantly collected in electronic automated databases and can be grouped as secondary data. They are presented in more detail in the following.
1. Administrative Databases
The provision of health care frequently generates digitised data such as claims data, hospital-based electronic data, and medication prescription records, collectively subsumed as “administrative database”. These are predominantly designed for billing and record-keeping purposes, and not primarily intended for research. A disadvantage of administrative data is the variation in size of the population, e.g., due to migration.
2. Claims Databases
For pharmacoepidemiologic research, claims data (predominantly claims to insurance companies for reimbursement) provide some of the best data on the prescription of medications on an individual basis. In claims processing, large amounts of data are generated and stored, detailing the services provided, often accompanied by supplementary information such as medical diagnoses and/or demographic information of individuals. It has to be recognised that different types of claims are not kept in the same database; e.g., pharmacy claims may be processed separately from the rest of the medical claims, which requires provisions for linking different databases.
3. Electronic Health Records (EHR)
An Electronic Health Record (EHR) (Masnoon et al., 2018) is defined as a longitudinal digital record of patient health information comprising all available data from different sources, including EMRs (see below). EHRs provide a 360-degree view of an individual’s health and wellness and also include data from patient portals or external laboratories and can be linked to further data sources. Data from EHRs are increasingly seen as a valuable data source for pharmacoepidemiology, pharmacovigilance, and clinical research requiring similar observational clinical data (Murray, 2014).
4. Electronic Medical Records (EMR)
EMRs are digital versions of the paper charts in clinician offices, clinics, and hospitals acting as a repository of patient health information and support capture and access to this information. EMRs provide administrative functionality and basic connectivity to enable tracking of patients and exchange of information. EMRs offer a higher level of detail compared to administrative data or patient/provider questionnaires. The EMR is based on real-time data, thus there is minimum latency period between the generation of data and its availability.
As with all secondary data EMR data are not specifically recorded for research purposes. They may be lacking entries of laboratory reports, progress notes, quality of life measurements, and information on health beliefs or health behaviours. The data contained in EMRs are typically complex, given the longitudinal nature and volume of information, and can be challenging for conducting statistical analysis. Multisite sources can be advantageous in terms of sample size, but there may be problems with data consistency and validity because documentation and coding practices (diagnostic information) or implemented functions may vary across the participating sites.
Despite limitations, EMR databases are nowadays widely used, often replacing paper medical records as the primary document (for further preference please see IMI BD4BO project). This facilitates the large data collection for pharmacoepidemiologic studies and, by cross-linking digital medical records, may enable researchers and physicians to monitor, among others, post-marketing safety. Some of the advantages are:
- The validity of the diagnosis data is superior to that in administrative databases.
- Cost of data collection is low - helpful when budget is restricted.
- The representativeness of research is enhanced by covering the entire population of a region.
- Large sample size permits examining rare outcomes and prescription patterns of infrequently used medications and list their uncommon effects.
- There is no recall and interviewer bias (they do not rely on patient recall or interviewers to obtain their data)
- They can potentially be linked to external electronic databases (e.g., birth and mortality records, claims), via a unique identification number, enhancing the capabilities and scope of research.
- They are useful when short-term effects of medications and objective, laboratory-driven diagnoses are studied; when there is limited time.
Examples:
The General Practice Research Datalink (GPRD) in the UK, has population-based anonymised longitudinal medical records from primary care data with over 15 million patients and has been extensively used for published research in the field of pharmacoepidemiology. https://www.cprd.com/
The German Pharmacoepidemiological Research Database (GePaRD) is based on claims data from statutory health insurance providers and currently includes information on about 25 million persons who have been insured with one of the participating providers since 2004. In addition to demographic data, GePaRD contains information on prescriptions, outpatient and inpatient services and diagnoses. Per data year, there is information on approximately 20% of the general population from all geographical regions of Germany https://www.bips-institut.de/en/research/research-infrastructures/gepard.html
5. Social media
Technological advances have dramatically increased the range of data sources that can be used to complement traditional ones and may provide insights into effectiveness and safety of interventions (see also Patient-Generated Health Data (PGHD) section). Social media is considered a sub-set of digital media and data exist in a computer-readable format as websites, web pages, blogs, vlogs, social networking sites, internet forums, chat rooms, health portals. This data is unsolicited and generated in real time.
The European Commission’s Digital Single Market Glossary defines social media as “a group of Internet-based applications that build on the ideological and technological foundations of Web 2.0 and that allow the creation and exchange of user- generated content. It employs mobile and web-based technologies to create highly interactive platforms via which individuals and communities share, co-create, discuss, and modify user-generated content.”
Social media are a heterogeneous data source. Social media has been used to provide insights into the patient’s perception of the effectiveness of medicines and for the collection of patient-reported outcomes. However, it must be acknowledged that the main purpose of most social media publications is different from e.g. reporting a suspected association between one specific health intervention and an adverse event (for further information see the IMI WEB-RADR European collaborative project which explored different aspects related to the use of social media data as a basis for pharmacovigilance and summarised its recommendations in Recommendations for the Use of Social Media in Pharmacovigilance: Lessons From IMI WEB-RADR (Drug Saf 2019;42(12):1393-1407)).
Indeed, one possible use of social media would be as a source of information for signal detection or assessment. Studies have evaluated whether analysis of social media data (specifically Facebook and Twitter posts) could identify pharmacovigilance signals early, but in their respective settings, found that this was not the case. However, a report from 2020 found that social media data actually could have indicated the outbreak of COVID 19 prior to the health system as certain key words were used much more frequently in posts (https://www.eurosurveillance.org/content/10.2807/1560-7917.ES.2020.25.10.2000199?crawler=true).
The rapidly evolving social media environment presents many challenges including the need for viable processes for selection, validation and study implementation. There is currently no defined strategy or framework in place to address concerns about generalisability and validity. Social media data can include slang, emojis, false IDs and duplicates, hidden messages (such as Twitter sub-hashtag) or comments intended to foster a false belief. However, more tools and solutions for analysing unstructured, heterogenous data and free text (natural language[1]) in large volumes (Big Data[2]) are becoming available, especially for pharmacoepidemiology and Drug Safety research, employing emerging techniques, using Artificial Intelligence (AI) (with its subfield of machine learning[3]), neural network architectures, deep learning. Social media platforms may potentially constitute an important resource for medical research, if their potential can be realised. However, there is also the possibility of negatively influencing research, e.g. young patients in a trial connected via social media might in a chat inadvertently discover they are in the same trial and run the risk of unblinding or compare outcomes and may conclude in which arm of the study they were.
There are various other sources of data employed in pharmacoepidemiology that do not correspond to any of the previous categories. Results of laboratory tests contained in laboratory collections may be used as surrogates to monitor the outcome of interest; monitoring systems, such as disease specific surveillance systems to monitor infectious disease outbreaks like seasonal influenza and tuberculosis, and national statistics, such as mortality (death certificate data) and statistics on hospitalisations. Data can also be found outside of healthcare systems. For example, sales of medical products could be obtained from a pharmacy or a group of pharmacies in a specific area. Also, sales data have been utilised as a method of syndromic surveillance to predict disease outbreaks.
[1] Natural Language Processing (NLP): Computer software that can analyse, ‘understand’, and generate language that humans use naturally. It can be used to extract key terms or phrases from bodies of unstructured text, providing insights faster than a human can.
https://www.microsoft.com/en-us/research/group/natural-language-processing/
[2] Big Data: High-volume, high-velocity, and/or high-variety information content http://www.forbes.com/sites/gartnergroup/2013/03/27/gartners-big-data-definition-consists-of-three-parts-not-to-be-confused-with-three-vs/#3f03b5ee42f6
[3] Machine Learning: A method of data analysis that uses algorithms that iteratively learn from data so that computers can find hidden information without being explicitly programmed where to look. http://www.sas.com/en_us/insights/analytics/machine-learning.html
1.4. Patient-Generated Health Data (PGHD)
Patient-Generated Health Data (PGHD) [1]
"The amount of health information generated digitally across socio-cultural domains is unprecedented." The Committee on Data for Healthy Societies [2]
With the rapid rise of digital health, encompassing electronic healthcare data sources, such as electronic health records (EHR) and administrative/claims databases and further advanced by the development of innovative, often mobile, digital health technologies, the landscape of health care and related research has changed considerably.
Without going into further detail, the following graph gives an overview of the basic components of digital health in the healthcare ecosystem.
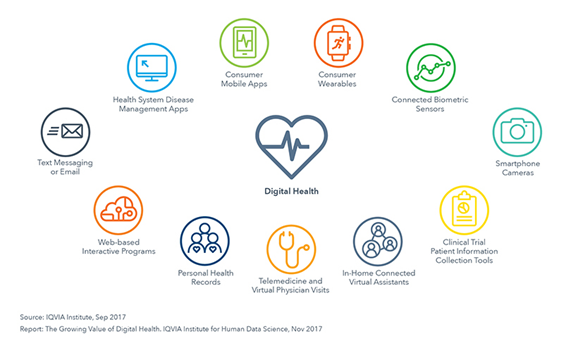
Figure 1: Non-comprehensive overview of digital health components.
This is accompanied by an emerging emphasis on patient centricity highlighting the growing importance of Patient (or Citizen/Person) Generated Health Data (PGHD) as a new data source which can be positioned on the intersection between the digital revolution and the patient-centred care movement. PGHD has made it possible for patients to capture, use, and share their health data in day-to-day settings and in real time with clinicians and researchers.
In the past, researchers often were limited to the data collected at the study site at regular intervals or from logs of data captured by patients and brought to the study site. With PGHD, new types of data are available in increased volume and frequency directly from patients using digital devices, both passively [4] and actively [5]. For example, health devices, such as connected glucose sensors, record data at short intervals or continuously, and they can transmit data directly and electronically to patients themselves and to clinicians and researchers.
Figure 2 highlights some of the sources and tools that facilitate collection of PGHD. There may be overlap between tools, such as when smartphones are used to complete online surveys, or their built- in sensors facilitate passively collected data.
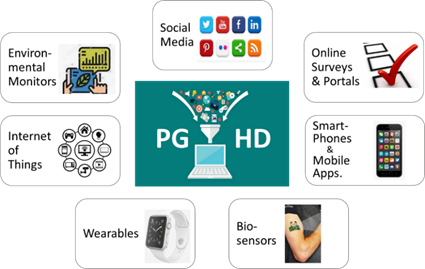
Figure 2: sources and tools for collecting PGHD (after Bourke et al.,2020)
At the population level, researchers can analyse large volumes of PGHD using artificial intelligence (AI) and sophisticated analytical tools, can interpret free-text (applying NLP), unstructured PGHD and link them with other health-related data. Through this process, researchers can identify and confirm association between a medicine and exposure, side effects and adverse events and predict and track the spread of infectious diseases or predict outbreaks (e.g., influenza) earlier and with greater accuracy than with traditional methods. The use of PGHD technologies to capture and transmit data electronically helps to simplify the research workflow: manual data entry can be replaced by electronic data transmission into a research Findings database, data checking for completeness and preparation for analysis can likewise be automated and the potential for human error during data entry can be minimised.
Specifically, for pharmacoepidemiology PGHD has the potential to address some of the existing challenges such as misclassification, representativeness, and missing information. It helps to gain person-centric insight not readily available in routine healthcare datasets and collect more and different variables, and with greater frequency/continuity than may be possible in clinical/research settings. In pharmacoepidemiology and epidemiology research studies, PGHD can be viewed as complementary, rather than a replacement of other data sources.
The following lists a number of possible advantages and challenges (not comprehensive) when applying PGHD data in healthcare and pharmacoepidemiologic research.
Advantages of PGHD
- empower the patient to track, change and improve health by enabling better self-management
- support healthcare professionals monitor and assist their patients, allowing real-time adjustments to treatment in response to patients' symptoms and physiology
- improve relationships and communication between patients and healthcare teams and support shared decision-making
- augment patient-driven quality of care assessment
- provide additional information to inform pharmacoepidemiologic research on:
o adverse events, especially non-serious events, their severity or their impact on patients' lives
o previously unassessed factors including patient-reported outcomes such as functionality, quality of life, pain or depression scales, as well as more accurate and precise information on timing and severity
o additional covariates (potential confounders and effect modifiers) which may not be present in EHRs, such as weight, smoking, alcohol consumption, as well as physical activity, sleep, mobility, location, diet and biochemistry (e.g., home-based blood glucose measurements).
Challenges of PGHD
- Imbalanced penetration among the entire population
- underdeveloped strategies for long term patient engagement
- data validity – e.g., devices to objectively measure medicines use
- data generalisability – especially relevant when using social media data
- concerns that receiving PGHD adds to clinical workloads and disrupts workflows contrary to expectations
- paucity of validated instruments, e.g., to measure patient reported outcomes.
- device standardisation and consistent accuracy
- high volume data is challenging to analyse because of its format or high frequency of measurement (e.g., continuous recording of heart rates or blood pressures).
- technical and privacy concerns, cybersecurity - can make linking disparate data sources problematic
- legal aspects such as informed consent and data security, (electronic-consent (e-consent))
- possible third-party involvement for encryption, pseudonymizing and anonymization for security of sensitive data.
- selection bias is possible if patient characteristics that underlie the ability to create PGHD (e.g., ownership of certain digital devices) are associated with both exposure and outcome of interest
Examples:
- built- in sensors in digital devices facilitate passively collected data, e.g., accelerometry to estimate hand tremors in people with Parkinson's Disease
- Smartphones: collection of unique types of PGHD, such as speech recordings in assessment of Parkinson's Disease severity
- Smartphones: frequency of social communication to monitor episodes of depression
- Digital Health Applications (DiGAs)[6], authorised by regulatory authorities are on the rise. A DiGA can not only include software but also devices, sensors (and other hardware such as wearables). A few examples from the DiGA directory of the German Federal Institute of Drugs and Medical Devices (BfArM)[7], the first regulatory authority to approve DiGAs, are given in the table below together with their indication (status 04/2021):
Name |
|
deprexis |
depressive episodes |
elevida |
Multiple sclerosis |
Kalmeda |
Tinnitus |
Rehappy |
Transitory cerebral ischemia |
somnio |
Insomnia |
zanadio |
Obesity |
[1] The following section on PGHD is mainly based on published information from the white paper of the ONC and the paper by Bourke et al.: Alison Bourke, William G Dixon, Andrew Roddam, Kueiyu Joshua Lin, Gillian C Hall, Jeffrey R Curtis, Sabine N van der Veer, Montse Soriano-Gabarró, Juliane K Mills, Jacqueline M Major, Thomas Verstraeten, Matthew J Francis, Dorothee B Bartels, Incorporating patient generated health data into pharmacoepidemiologic research Pharmacoepidemiol Drug Saf (Pharmacoepidemiology and drug safety) [2020, 29(12):1540-1549]
[4] Passive data collection: people making no or little additional effort, such as wearing/ carrying a device, or by re-using person-generated data previously recorded for non-health purposes (Examples: data from sensors in wearable and mobile devices (e.g., accelerometers, gyroscopes, GPS) or from social media (i.e., person-generated data that was initially recorded for non-health purposes providing information on exposure, confounders or outcomes).
[6] According to the German Regulatory authority (BfArM) a DiGA is a CE-marked medical device that has the following properties:
- Medical device of the risk class I or IIa (according to the Medical Device Regulation (MDR) or the transitional Medical Device Directive (MDD)). Information as to "when is an App a medical device?"; can be found here .
- The main function of the DiGA is based on digital technologies.
- The medical purpose is mainly achieved by way of its digital function.
- The DiGA supports the recognition, monitoring, treatment or alleviation of diseases or the recognition, treatment, alleviation or compensation of injuries or disabilities.
- The DiGA is used by the patient alone or by patient and healthcare provider together.
These requirements are defined in Section 33a of the German Social Code Book.
[7] DiGA directory of the German Federal Institute of Drugs and Medical Devices (BfArM): BfArM - Digital Health Applications (DiGA)
1.5. Health Database networks
Pooling data across different databases affords insight into the generalisability of the results and may improve precision. A growing number of studies use data from networks of databases, often from different countries.
From a methodological point of view, research networks have many advantages over single database studies:
- Increase the size of study populations which facilitates research on rare events, medicines used in specialised settings, or when the interest is in subgroup effects.
- Shorten the time needed for obtaining the desired sample size and speed-up investigation of medicine safety issues or other outcomes in case of primary data collection.
- Benefit from the heterogeneity of treatment options across countries, which allows studying the effect of different medicines used for the same indication or specific patterns of utilisation.
- May provide additional knowledge on the generalisability of results and on the consistency of information, for instance whether a safety issue exists in several countries. Possible inconsistencies might be caused by different biases or truly different treatment effects revealed in the databases. Addressing case definitions, terminologies, coding in databases and research practices by experts from various countries may help to increase consistency of results of observational studies.
- Allow pooling data or results and increase the amount of information gathered for a specific issue addressed in different databases.
Different models can be applied for combining data or results from multiple databases. A common characteristic of all models is that participating partners maintain physical and operational control over electronic data and therefore the data extraction is always done locally. However, differences exist in the following areas: use of a common protocol; use of a common data model (CDM); and where and how the data analysis is done.
Use of a common data model (CDM) implies that local formats are transformed into a predefined, common data structure, which allows similar data extraction and analysis across several databases. Sometimes the CDM imposes a common terminology as well, as in the case of the OMOP CDM. The CDM can be systematically applied to the entire database (generalised CDM) or to the subset of data needed for a specific study (study specific CDM). Initially used in the US, the OMOP has since been applied in Europe, specifically as part of the Innovative Medicines Initiative (IMI) European Medical Information Framework (EMIF) and European Health Data Network (EHDEN)[1]. In the EU, study specific CDMs have generated results in several projects, but experience based on real-life studies is still limited.
The growing use of health database networks has led to development of new analytical methodologies through the elaboration of large volumes of heterogeneous data (so called Big Data), as done by the Observational Health Data Sciences and Informatics (OHDSI[2]), which developed methods and tools for building network infrastructures. Similarly, the IMI Protect initiative showed how it is possible to conduct analysis on multiple databases, through the adoption of common protocols rather than through analysis of centralized data. Moreover, EMA has coordinated a network of centres of pharmacoepidemiology and pharmacovigilance (EnCePP) consisting of about 200 public institutions and Contract research organization (CRO) involved in activities related to pharmacoepidemiology and pharmacovigilance. The use of research networks in drug safety analyses is well established and a significant body of practical experience exists. By contrast, no consensus exists on the use of such networks, or indeed of single sources of observational data, in estimating effectiveness. In the context of database networks used for pharmacovigilance, two are the initiatives of reference implemented internationally: Sentinel (FDA)[3] and EU-Adr (EU)[4].
Other international networks have been established, with the aim of increasing the power of the post marketing studies on medicines and vaccines, including Aritmo, Safeguard, Advance, Sos, Euromedicat[5] in Europe, Cnodes in Canada and Asian pharmacoepidemiology network (Aspen) in Asia and Australia.
[1] EHDEN is a European IMI five-year project that builds on the EMIF’s work by creating a federated network of Real World Data (RWD) sources. It aims to harmonise approximately 100 million EHRs in the European Union by utilizing the OMOP CDM. It will focus mostly on population data sources and outcomes-based research for validation.
[2] Ohdsi https://ohdsi.org/
1.6. Data Privacy considerations for the use of Big Data
Health data are seen as sensitive data not only by the patients/citizens but also by almost all health authorities. Thus, it is important to ensure compliance with the respective regulation, e.g. the General Data Protection Regulation (GDPR) in Europe (https://gdpr.eu). The GDPR was put into effect on May 25, 2018, to create a data privacy regulation framework across Europe. Though it was drafted and passed by the European Union (EU), it imposes obligations onto organisations anywhere, so long as they target or collect data related to people in the EU. The GDPR will levy harsh fines against those who violate its privacy and security standards, with penalties reaching into the tens of millions of euros. While the GDPR also tries to privilege the use of health data for research, this area is still fragmented across Europe as the countries interpret the respective clauses differently when transferring the GDPR into more specific national regulation.
The overall aim is to prevent the identification of individuals using the collected data. This is getting increasingly challenging, as with more and more -omics data becoming available the use of these data in combination with other data from major studies or registries carries the risk that an individual patient becomes identifiable.
Several approaches are currently followed to protect the patient’s personal data while on the other side allowing best use of the data for future research and improvements of healthcare:
1. De-facto Anonymisation
Pooling the patient data in one database gives the highest flexibility in using
the data. On the other side this approach would allow the identification of
individual patients if all patient data are transferred into the central
database. To address this challenge the IMI project HARMONY has developed a so-called
de-facto anonymization concept. This describes how the original EHR data of a
patient should be stripped from some key data to make it impossible with
reasonable effort to identify an individual while keeping the dataset complete
enough to allow high quality research. This approach has been evaluated by data
privacy experts as GDPR compliant. For details see: HARMONY Anonymization Concept
Reconciles Data Quality, Safety, and Privacy - HARMONY Alliance
(harmony-alliance.eu)
2. Federated Data Networks
Another approach to pool data from different sources are the so-called
Federated Data Networks. In this case the data stay in their original place and
access is controlled by the respective database owners. This allows linking of
the databases and harmonised searches across the different data sources harmonisation
of the databases and the use of a common data model (see above under 8.5). A
search / question will then be sent to all network partners, which will then
run the search in their database and the search results from all partners will
be combined. In this case no patient identifiers will be transferred to the
coordinating entity and data privacy will be ensured. For example, this
approach is used by the IMI project EHDEN which has recently supported OHDSI in
a virtual studyathon[1] to
inform healthcare decision makers in response to the COVID-19 pandemic (COVID19 Study-a-thon.)
3. Platforms empowering each patient to control access to their health data for different purposes and by different users.
There are first examples of not-for-profit organisations running health data platforms which allow participating patients to have total control and self-determination in their digital health environment. They aim at linking the different sources hosting the data of a patient and giving the patient full control of deciding for which purpose and to whom he/she wants to give access to the data. Examples are the platform data4health of the Hasso Plattner Institute in Potsdam, Germany (www.data4life.care/en ) or the platform MIDATA in Zurich, Switzerland (www.midata.coop/en/home), which links patient data on a regional and national level with full control by the participating patients.
[1] A studyathon is a multi-day web event bringing together a comprehensive and diverse range of experts to have an uninterrupted deep-dive on a specific disease or healthcare topic.