Principles of Sample Size Calculation
Site: | EUPATI Open Classroom |
Course: | Statistics |
Book: | Principles of Sample Size Calculation |
Printed by: | Guest user |
Date: | Saturday, 27 July 2024, 2:58 AM |
1. Introduction
(This section is organised in the form of a book, please follow the blue arrows to navigate through the book or by following the navigation panel on the right side of the page.)
In a clinical trial, the objective is to obtain information about the effect of a treatment in a certain patient population who are likely to benefit from that treatment. However, the researchers cannot administer this treatment to the entire population. It would not be realistic for ethical, financial and often logistical reasons. Therefore, the clinical trial will be conducted only on a sample from the population of patients. This population sample should be representative of the whole population in order to allow the generalisations of the clinical trial findings.
2. Why Sample Size is Important?
2.1. Components of Sample Size Calculations
Component |
Definition |
Alpha (α) (Type I error) |
The probability of falsely rejecting the null hypothesis (H0) and detecting a statistically significant difference when the groups in reality are not different, i.e. the chance of a false-positive result. |
Beta (β) (Type II error) |
The probability of falsely accepting H0 and not detecting a statistically significant difference when a specified difference between the groups exists in reality, i.e. the chance of a false-negative result. |
Power (1-β) |
The probability of correctly rejecting H0 and detecting a statistically significant difference when a specified difference between the groups in reality exists. |
Minimal clinically relevant difference |
The minimal difference between the groups that the investigator considers biologically plausible and clinically relevant. |
Variance |
The variability of the outcome measure, expressed as the Standard Deviation (SD) in case of a continuous outcome. |
Abbreviations: H0 – null hypothesis; the null hypothesis states that compared groups are not different from each other). SD – standard deviation.
2.2. How To Calculate the Sample Size for Randomised Controlled Trials
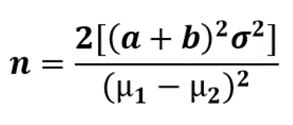
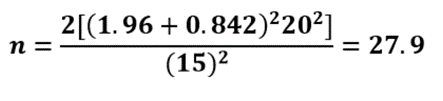
3. Sample vs Population
4. Sample Size Calculation
Sample size calculation is an essential part of the design of a clinical trial. The size of the study should be adequate in order to generate conclusive results. Calculating the appropriate sample size requires feedback on various aspects of the trials, such as the study design, the tested hypotheses, the targeted study power and the type I and II errors.5. What Drives a Sample Size Calculation?
There are 5 key drivers in sample size calculations.5.1. The Design of the Clinical Trial
A trial with only one or several experimental treatments arms in a Phase II setting will require a different approach from a randomised comparative Phase III trial. Furthermore, the sample size needed for a study depends on the assumption of the size of the difference expected between the two treatments being studied. In a study where a large difference between the treatments is assumed, the difference should be observable in a smaller sample, whereas a larger sample size is needed to detect a small difference between two treatments. The situation becomes more complex when more than two treatment groups are planned since there is no longer one single clear alternative hypothesis. A test strategy must be defined upfront and adequate measures applied to maintain the overall type I error.5.2. The Choice of the Primary Endpoint(s)
- Binary vs. continuous: binary indicates whether an event has occurred (occurrence or relief of symptoms), while continuous represents a specific measure or count (e.g., blood pressure).
- Landmark: its goal is to have a fixed time (time-to-event) after the initiation of the treatment, where analysis of survival can be conducted.